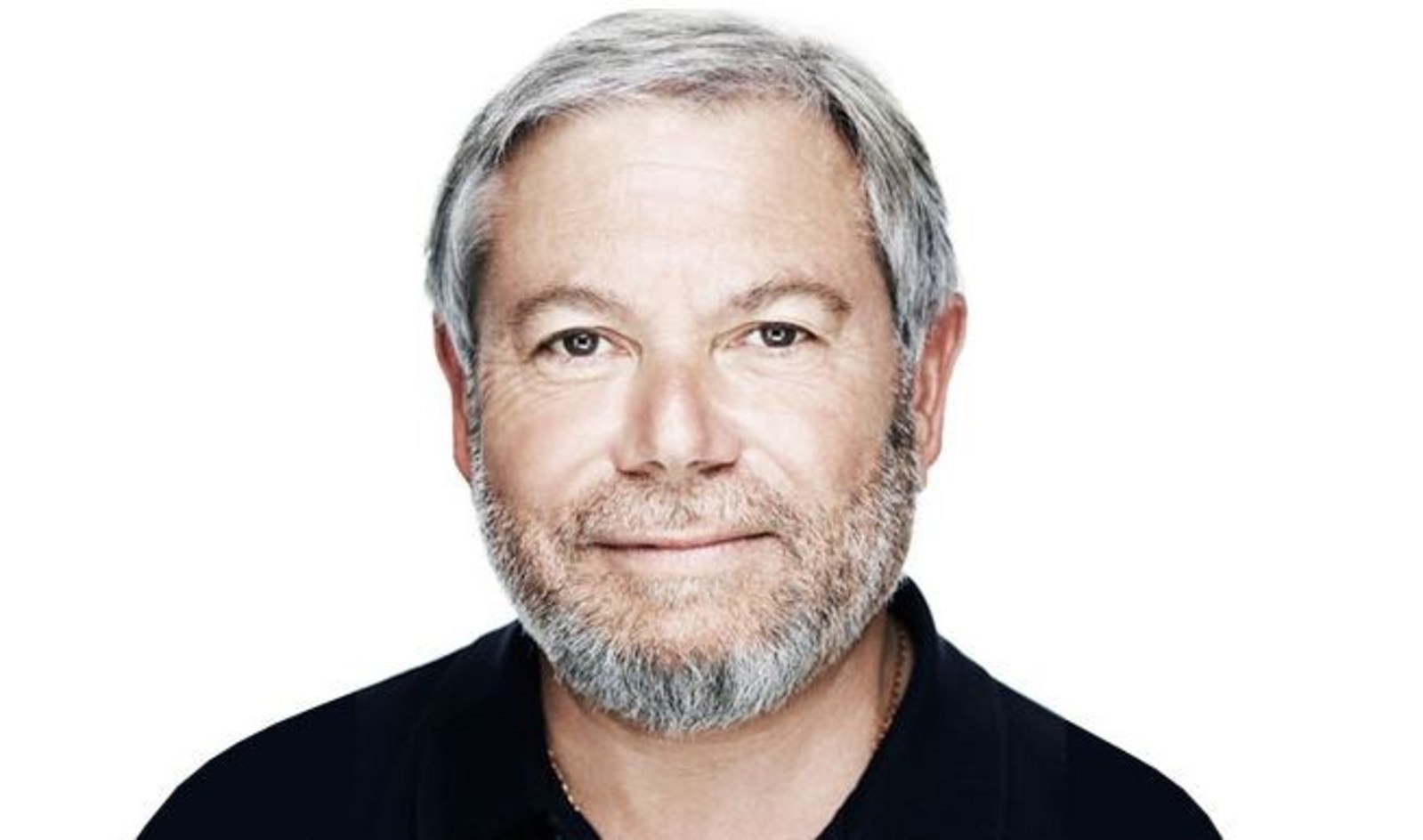
Printing parts seems to be an easy process when the manufacturer has all the tools (hardware, software and materials) or when he is given access to them. However, understanding what is happening inside the 3D printing process is not that easy. As a matter of fact, additive manufacturing involves a wide range of complex variables that need to be controlled in order to achieve a suitable level of accuracy and lightweighting in printed parts.
This quest for lightweighting is prompting a new way of thinking about design and involves technologies such as artificial intelligence that generate multiple design solutions for the automotive industry.
Techniplas, a supplier to BMW, Daimler, Ford, and Fiat, has been combining 3D printing and AI to make parts up to 47% lighter. Using illustrations from the company’s own experience, Techniplas Digital CEO Avi Reichental , demystifies the complexity of AI in manufacturing processes.
Technologies that enable lightweighting
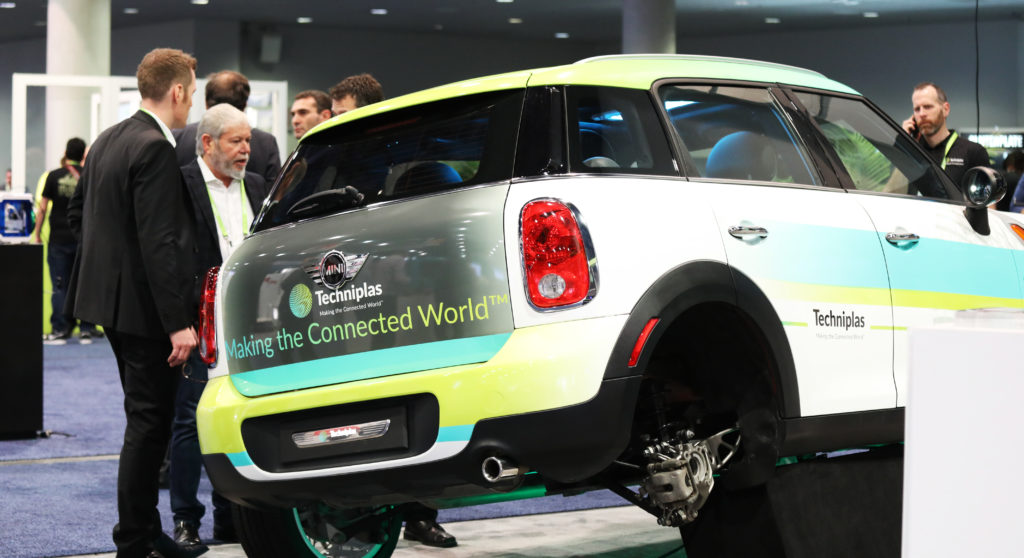
Many companies consider metal additive manufacturing as the first lightweighting enabler. Indeed, this 3D printing technology enables to achieve performance optimized parts that do not need too much materials.
However, Avi is looking at the first stage of the manufacturing process, which would be a crucial one in production: software. For Techniplas Digital CEO, the key to lightweighting (in a way that can reduce the material content) is generative design (GD).
Even though a standard definition of the concept does not exist yet, experts tend to describe it as a technology that takes advantage of mathematical calculations to assist in the design process. GD is often considered either as a broad term encompassing topology optimization (TO) or as a distinct technology.
“Companies like Autodesk can use the combination of generative design, topology optimization to fully optimize the part.” [The combination of these technologies would lead to efficient geometries, and would aim] to not only reduce weight but to increase performance”, said Avi Reichental.
This concept of topology optimization (TO) has been used in industries for decades, with the only goal to optimize distribution of materials in a design space. TO structures are complex in nature, therefore they are not often compatible with other manufacturing processes. AM instead, easily processes complex structures, which results in shorter product development time when combined with TO.
Speaking of the automotive industry in particular, Avi states: “professionals worry about fuel efficiency, safety and performance; and the combination of generative design together with AM is providing a key to unlocking significant weight production and performance improvement in automotive.”
Until now, it still hard to determine at what level AI comes into play.
How can Artificial Intelligence impact Additive Manufacturing?
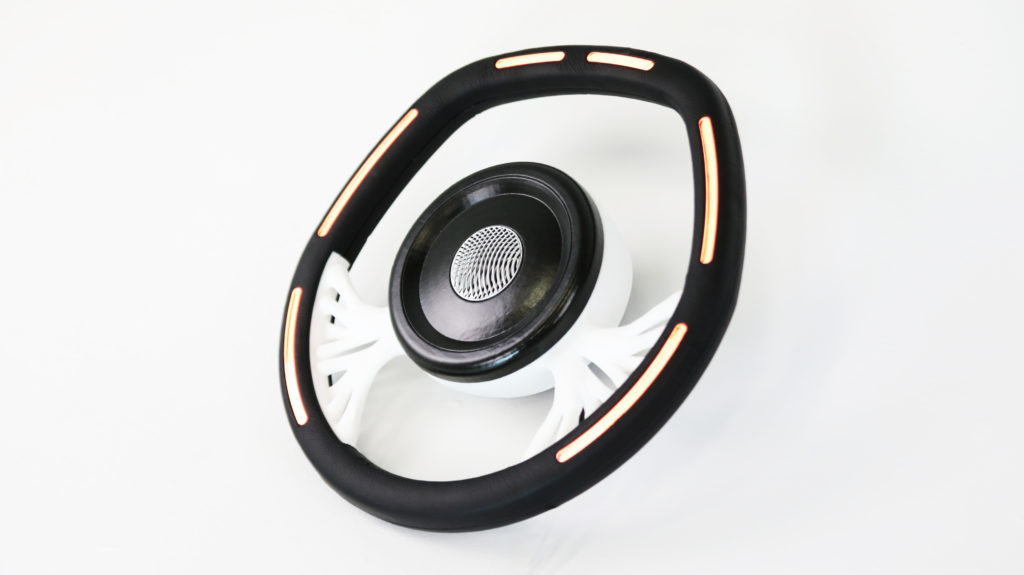
As mentioned earlier, AM requires a number of variables that need to be monitored to achieve an appropriate level of accuracy and lightweighting in printed parts. Professionals therefore apply trial and error tests for finding the perfect design of support structures. However, this solution takes a lot of time.
Generative design and testing are then combined to improve printing efficiency and cost reduction. AI on the other hand, is used to create intelligent service-oriented production processes for the industry.
According to Avi, a few applications of AI in AM can already be observed in the market. They include:
- “Intelligence real-time monitoring of 3D Printers used to improve process and traceability over time;
- The ability to provide predictive and prescriptive analytics to operators that are running 3D Printers;
- The optimization of capacity utilization for companies that are using farm of 3D Printers. [A 3D printer farm may impose some constraints to humans who will have to control 3D printers, monitor them constantly in order to ensure they run smoothly.]
- The final one is to marry the entire workflow from design to manufacturing with the best geometry to print or to optimize.”
These applications show how AI can impact AM in a significant way. They also show that, for now, enhancing design and improving the efficiency seem to constitute the most important use cases for AI in AM. However, when it comes to lightweighting, only one question remains:
What differences can we bring between the simple use of 3D printing to lightweight parts and the combination of 3D Printing and AI?
“If you use 3D Printing alone to alleviate parts, you are completely at the mercy of training and capability of engineers. They have experienced lightweighting and topology optimization and they can try to optimize geometry as much as possible if they have the required tools in their hands. What we have learned from the automotive companies Techniplas is working with, the outcome is limited to the training and experience of the design area.
Whereas, if we use AI and TO, we can cut most of the labour intensity, eliminate the variability of the design outcome and come up with very efficient redesigned parts that are up to 20 or 50% lighter, and generate a geometry that is ready for 3D printing.
If we just look at AM today with AI and generative design, it is not enough to achieve an acceptable production process. When we look at AM together with generative design, TO and AI, it becomes a very intelligent and capable workflow that can repeatedly reduce weight while creating optimized parts that can be used in demanding industries”, explains Avi.
One of the biggest challenges in additive manufacturing is the need for greater precision and the ability to reproduce 3D-printed parts. Avi’s explanations show that AI is a key tool for the automotive industry where exact geometric dimensions are crucial to enabling vehicles operating safely and reliably. Even though a few examples have been taken in the automotive industry, we strongly believe AI can be used in other demanding industries where manufacturing processes require hundred-million-dollar investments (e.g. aerospace).
In the meantime, before industries fully take advantage of these technologies, a few challenges need to be overcome; the most important one being education.
Indeed, generative design is a technology that is still in an early stage of development, which means that engineers certainly come across certain challenges.
Furthermore, speaking of challenges related to the automotive industry, Avi adds the importance to understand design cycles of automotive companies, the qualification of AM processes for automotive production, and the economic perspective as compared to injection moulding.
Final thoughts
While professionals still question the importance of AI in AM, companies such as Techniplas, that are at the forefront of innovation demonstrate that AI and machine learning foster the development of generative design technology. AM on its side, plays a key role in driving the adoption of this technology.
However, with improvements that still need to be made, expect the combination of AI, generative design, TO, and AM to be the greatest strength in tomorrow’s manufacturing.
For further information about 3D Printing, follow us on our social networks and subscribe to our newsletter!
Would you like to be featured in the next issue of our digital magazine? Send us an email at contact@3dadept.com
//pagead2.googlesyndication.com/pagead/js/adsbygoogle.js (adsbygoogle = window.adsbygoogle || []).push({});