Despite the capabilities of additive manufacturing, producing parts with only a trial-and-error approach remains time consuming and expensive. Indeed, you will never know the number of batch productions you are going to launch before obtaining the desired parts. Furthermore, there are so many reasons why an error may occur, errors that go beyond the simple design and manufacturing of the parts, that it has become crucial to ensure optimal process conditions within machines, processes and more. A Digital Twin (DT) technology might be a potential solution that can be leveraged to overcome many issues in additive manufacturing but the lack of thorough understanding of the DT concept, framework, and development methods constitute key factors that slow down the development and integration of such technology across AM production environments.
The lack of understanding of DT may often come from the assimilation to simulation technologies. The fact is, it is more than just “simulation”. A simulation process replicates what could happen to a product during the manufacturing process whereas a digital twin duplicates what is happening to an actual specific product in the real world. Interestingly, the DT concept goes beyond what may happen to a physical product, to encompass the prediction of production and performance within specific environments. DT therefore refers to a virtual representation of a physical product or process based on a computer program. The latter uses real world data to create simulations that can predict how a product or process will perform.
In manufacturing, a DT is a virtual copy of a real-world component in the manufacturing process. This means that it uses inputs from a real-world component to mirror the real part’s status, functionality, and/or interaction with other devices.
In additive manufacturing in particular, the greatest adoption of ‘digital twins’ would be tied to the design and manufacturing phase – according to Duann Scott, Consultant & Founder of Bits to Atoms. “I have only seen performance in testing during the design iteration phase, less so in the performance of a manufactured part over time”, he states.
While any industry that fabricates products is likely to use digital twin technology at a certain point, our focus in this feature will be to understand how the concept is applied within an AM production environment. To do so, the first step consists in understanding the potential of this technology and its framework.
What framework applies to the use of DT in manufacturing?
No matter what manufacturing process is utilized, the first step required to integrate a DT technology is to create or provide access to virtual representations of the products, machines, and environments that organizations design, manufacture or operate.
This integration therefore relies on the incorporation of CAD or 3D Modeling Tools, IoT/Connected Devices, Game Engines, version control, multi-physics simulation, data analytics, and machine learning capabilities to demonstrate the impact of design changes, usage scenarios, environmental conditions, and other variables; the ultimate goal being to remove the need for physical prototypes, decreasing development time, and enhancing the quality of the finalized product or process.
“To ensure accurate modelling over the entire lifetime of a product or its production, digital twins use data from sensors installed on physical objects to determine the objects’ real-time performance, operating conditions, and changes over time. Using this data, the digital twin evolves and continuously updates to reflect any change to the physical counterpart throughout the product lifecycle, creating a closed-loop of feedback in a virtual environment that enables companies to continuously optimize their products, production, and performance at minimal cost”, experts from Siemens Digital Industries Software said.
Furthermore, the convergence of these technologies indicate the various ways DT can be used in manufacturing environments:
- As explained at the beginning, to avoid going through a trial-and-error process, to test a new product, digital twins can be leveraged to test out updated configurations while reducing the risk of costly miscalculations. Ingersoll Machine Tools explored the DT concept this way to achieve better ROI targets.
- By planning out and testing new production lines using DT, operators can easily find potential issues and areas to optimize before they create the physical production line. In this case, DT helps to enhance a system design. That’ exactly what Shell did for its Singapore-based Pulau Bukom Manufacturing Site.
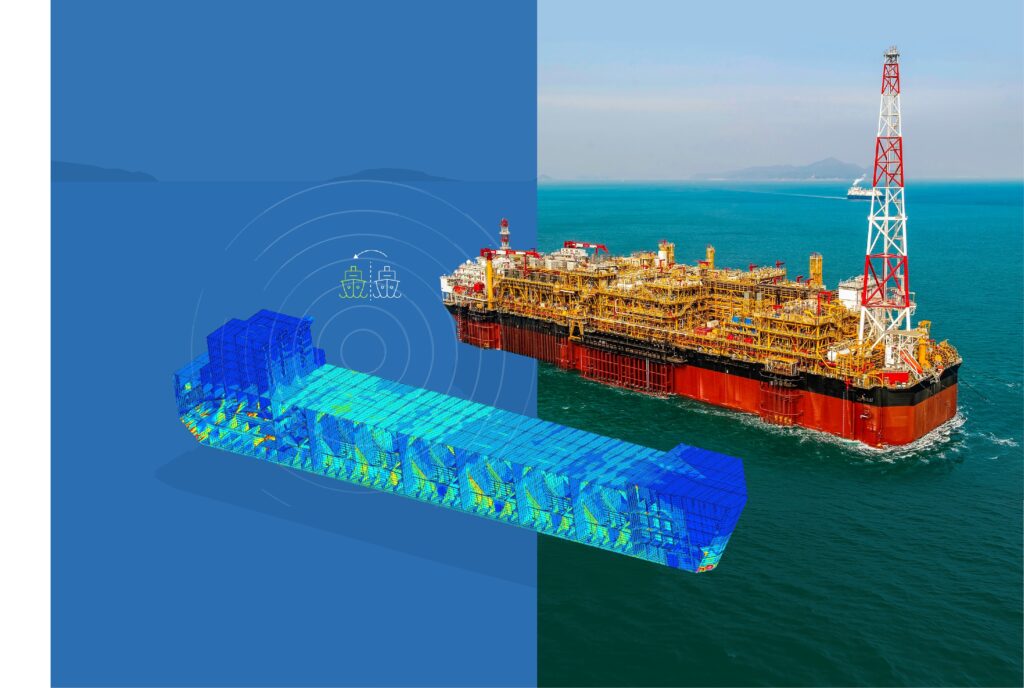
- Innogy Ventures and Shell Ventures Back Predictive Digital Twin Pioneer with $10 Million Investment
This use of DT is gradually seen in fields such as maintenance, repair, overhauls, etc. Decision Lab Ltd and Siemens have developed a digital twin, ATOM, which emulates the global maintenance repair and overhaul (MRO) operations of Siemens’ aero-derivative gas turbine division. The Agent-based Turbine Operations & Maintenance (ATOM) model is driven by live data already available within the supply chain. It can visualize fleet and maintenance facility operations, capture and predict key performance indicators (KPIs) of the system, and even quickly run a virtual and detailed scenery to help make decision of investment.
- Given the amount of vital information operators often collect from their machinery – information related to humidity, motion, vibration, etc.- the combined used of IoT connected devices and digital twins can facilitate their incorporation into a comprehensive view of a system, complete with real-time data. This way, if a component begins to develop an unexpected behaviour, the manufacturing team will be aware of it before it has the chance to halt production or become a hazard. At this stage, given the specifications of each manufacturing machine that can be used in a production environment, it’s often easier for each OEM to develop digital twin solutions that may meet the specific needs of their technology. That’s what machine manufacturer Solukon did with the development of its Digital Factory Tool – that it is upgrading following a partnership with Authentise.
- According to software company Perforce, with digital twin technology, manufacturers can provide augmented reality (AR) programs to maintenance technicians. Through AR glasses, technicians can view the most up-to-date models of the machine laid over the one in front of them. This ensures they always have the right specs as they need them.
Several projects – that ambition to demonstrate how the DT concept can serve the AM industry – are currently in progress. Furthermore, the incorporation of the aforementioned technologies (CAD or 3D Modeling Tools, IoT/Connected Devices,Game Engines, version control, multi-physics simulation, data analytics, and machine learning capabilities) definitely has a role to play in the construction of a digital twin of AM hardware, software, and related technologies, but there is no consensus yet on the right technologies to leverage for each manufacturing device. On the other hand, most of these technologies raise their share of challenges that must be overcome.
Major challenges to overcome to create a DT of AM led by dynamic data-driven application systems
The concept of DT is challenging at different levels and since it mostly makes sense to deploy it at scale, the first barrier AM teams often face is data.
According to Scott, “the most challenging aspect of implementing and deploying a digital twin, is that the data typically needs to flow through different software and hardware vendors, so becomes less of a digital twin, and more of a digital quintuplet unless there is a unified file format that meets the needs of every software. Having sometimes competing companies collaborate on behalf of the customer to ensure the data is communicated without loss or fragility due to software or firmware updates can be very challenging and time consuming.”
Interestingly, other major issues often navigate around this problem of data. They include a real-time digital representation of the physical domain in additive manufacturing, database and models, IoT and machine Learning.
To realize the real-time digital twin of AM, it’s crucial to obtain the heat transfer and thermal distribution, melt-pool solidification, residual stress and distortion, structures and properties of the 3D printed parts as well as the operation conditions of the machines. Normally, the appropriate sensors could obtain some data such as the temperature distribution.
“A lot of information needs to be computed and simulated, which is very time consuming based on the current available computing capabilities. Currently, the prediction of temperature distribution inside an AM part that is being printed with non-proprietary mesh-based finite element models will take at least several hours, if not days”, a research reads.
Furthermore, the digital twin of AM needs plenty of data to train the model to improve the accuracy of the model. The data could come from experiments, sensors and numerical simulation. However, to collect and classify a sufficiently large volume of useful data is intractable, and usually the concepts of the Internet of Thing (IoT) and cloud computation may be necessary. To date, in actual production, the big data obtained from a product’s lifecycle is still isolated, fragmented, and stagnant because the convergence between the product’s physical and virtual space is lacking, which made it difficult to utilize the data, the same research explains.
Nevertheless, a nuance is brought for additive environments that involve modeling. Such an environment requires a lot of models, therefore too much data to verify them. However, to reduce the “computational burden”, there is a great need for a temperature-dependent thermophysical properties database for commonly used engineering alloys.
On another note, to adopt a DT in AM, an effective Internet of Things system is of paramount importance for each part of the system to be linked. As seen with the Solukon Digital Factory, a smart connection for the sensors, equipment and system should be effectively achieved. Massive amounts of data in the additive process will also play a key role in the creation of a digital twin that interacts with the cyber domain by means of the Internet of Things. For this operation to run smoothly, OEMs need to ensure there is a link between existing brownfield systems and their data.
As for machine learning, this technology is important as the DT depends on the concept of data driving.
Through learning based on data gathered from various resources such as simulation, experiments, literature, machine learning could make reliable predictions on microstructure, properties and defects. This technology could extract helpful information and relationships from data instead of phenomenological guidance or explicit programming; and the solution of complex equations from physical and math problems based on phenomenological understanding could be avoided. Thus, the calculations are rapid. The quality and volume of data will decide the accuracy of the predictions, the research lays emphasis on. The good news is, it is not difficult to build machine-learning programs once we have well-tested, user-friendly, and reliable algorithms.
So, how do we foster the adoption of DT across AM production floors ?
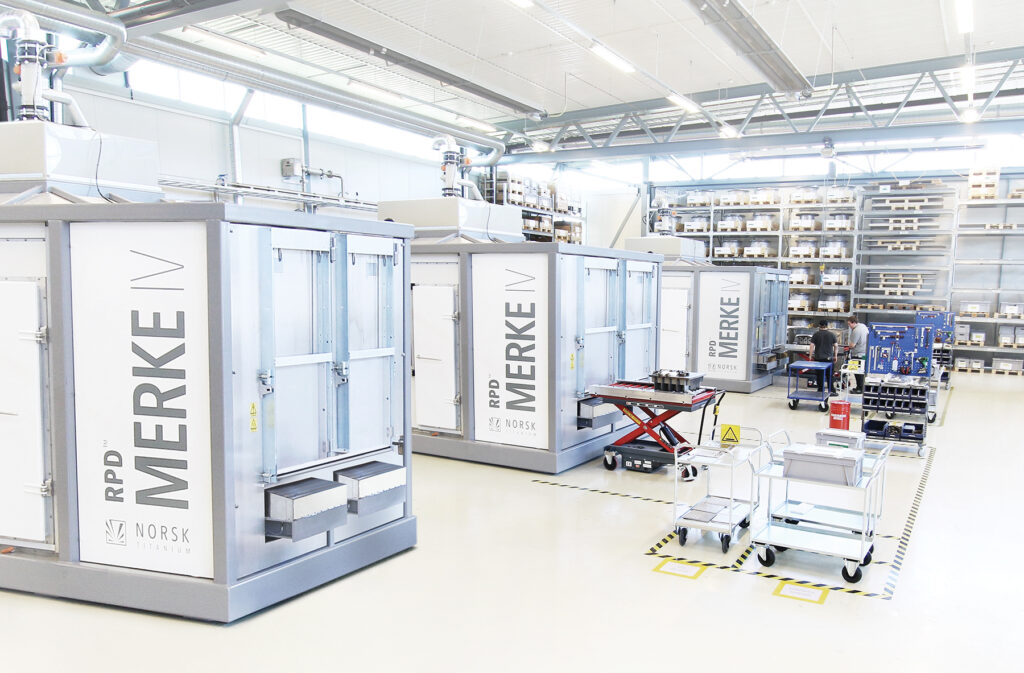
It’s really weird to say, but the DT technology can be both complex and simple at the same time. Its complexity lies in the challenges to overcome – most of them revolving around data -, while its simplicity lies in the range of possibilities it allows for the prediction of products, production and performance.
Amid the short list of software companies/collaborations that currently develop and commercialize DT solutions for the AM industry, one notes Siemens NX, Autodesk (the Additive Simulation Extension available in Netfabb and Fusion 360), Authentise and Nebumind, Intellegens & Ansys as well as Vertex.
Moving forward, “the quickest way to adopt digitization of the manufacturing process is to empower those working on the factory floor to implement connectivity and analyze the results. We are seeing new waves of digital tools that make this possible to implement, with less reliance on IT departments and information officers which may slow things down in getting buy-in. It is often said that every time a decision has to go ‘up the chain’ you half the chance that it will be understood or approved. By enabling those closest to create and connect, the time and cost of adoption is lower, and the ability to tune the connectivity by enabling those closest to create and connect, the time and cost of adoption is lower, and the ability to tune the connectivity quickly [makes it possible to leverage data in the most efficient way within] the manufacturing process”, Scott concludes.
This article has first been published in the May/June edition of 3D ADEPT Mag. Remember, you can post jobopportunities in the AM Industry on 3D ADEPT Media free of charge or look for a job via our job board. Make sure to follow us on our social networks and subscribe to our weekly newsletter : Facebook, Twitter, LinkedIn & Instagram ! If you want to be featured in the next issue of our digital magazine or if you hear a story that needs to be heard, make sure to send it to contact@3dadept.com