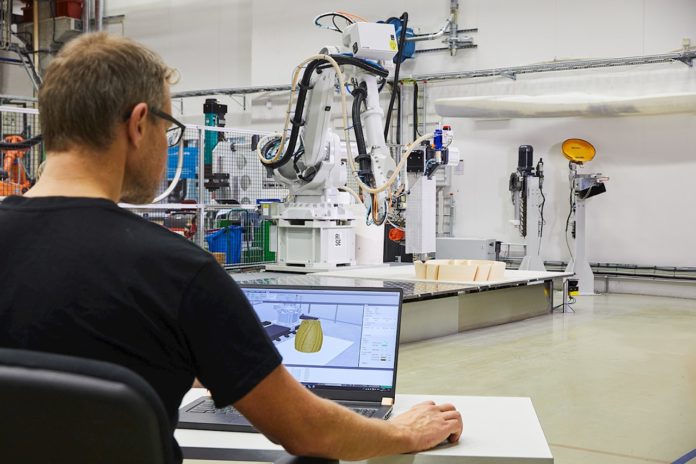
When early users of Additive Manufacturing technologies started leveraging these technologies, the most important aspect, if not the only one, was to determine if those technologies could effectively achieve rapid prototyping. As users’ needs have evolved towards series production or even mass production, technologies’ capabilities also need to be adapted. Automation comes into play and becomes a pivotal goal to reach, to truly enable series production of 3D printed parts, but the route towards true automated additive manufacturing production is often strewn with pitfalls.
Needless to say, automation is a vast topic. In the manufacturing field, where most of the tasks were historically done by hand, automated manufacturing has helped operators to rely on a number of computerized control systems to run equipment in a facility. In an industrial additive manufacturing setting especially, one quickly observes that depending on their needs, facilities can implement automation strategies at various levels of production – no matter what AM technology is harnessed – : software, pre-processing, manufacturing or even post-processing.
Despite its crucial role in manufacturing, software automation in additive manufacturing is often the part that is least understood by users of additive manufacturing, which is why we’ve decided to explore it in this exclusive feature.
What drives software automation in additive manufacturing? What are the key stages that are taken into account to advance Additive Manufacturing Software Automation? Where are the current needs/challenges? This article aims to address these questions with examples taken on various types of AM technologies.
Benjamin Schrauwen, CEO of software company Oqton has also been invited to share key insights into this topic.
What drives software automation in Additive Manufacturing?
In traditional manufacturing, automation is used to increase efficiency and speed. Not only do machine manufacturers and software developers want to bring those advantages to additive manufacturing, but they need to bring them at a more advanced level in order to justify the use of AM technologies in production. This explains the increasing number of collaborations between machine manufacturers and software developers, software developers and material producers, or even between software companies with various expertise.
As you will have understood, software is the backbone for any attempt at automation. To understand the various levels that often require the attention of software companies, it’s important to categorize the different types of software solutions that can be leveraged in additive manufacturing.
We’ve identified six main types of software in the Additive Manufacturing industry: Design (CAD), Part identification, Simulation (CAE); Processing (CAM), Workflow (ERP/MES), and QA & Security.
When asked what are the areas that would require automation the most, Benjamin Schrauwen, CEO of Oqton, a software company that is solving manufacturing challenges with an AI-driven factory operating system, explains:
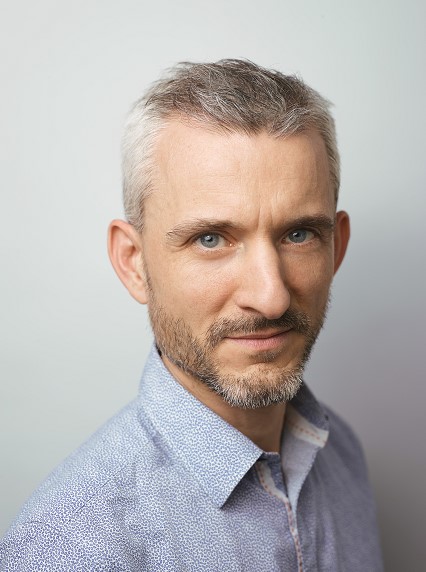
“Any manual, repetitive task that is currently performed by manufacturing engineers should and can be automated. This way, we can increase efficiency and productivity while freeing up their time to focus on value-adding work. This is also known as ‘end-to-end automation’ of the workflow.
We see this as very important, for example, dental and polymer AM service providers who produce high quantities of mass customized and personalized parts. Manufacturing Execution Systems (MES) is a big area for automation, too: we believe an intelligent way of scheduling is the way to go. This would be completely automated based on printer, material, and people capacity.
While it highlights some of the key stages that are currently taken into account to advance software automation strategies, Schrauwen’s explanations also reveal that what currently drives software automation in AM has moved beyond the reach of the software that operates the equipment.
Indeed, industrials have no choice but to consider an automation strategy at the software level, if they want to achieve mass production – therefore, save time, costs and design resources -.
Although that’s a concern tackled by machine manufacturers, an automation strategy at the software level is prevalent, when we know mass production with AM inevitably requires to achieve consistent and repeatable parts.
Most importantly, as Schrauwen’s notes, “any manual, repetitive task that is currently performed by manufacturing engineers should and can be automated”. This means that, the first step in deciding where one should focus their automation strategy consists in identifying those tasks and assessing the stage that will require an immediate action.
The different levels where value and complexity can be enhanced through software automation
Not surprisingly, manual tasks can start way before the manufacturing process itself. They consist in but are not limited to entering data into systems, replying to customer requests, calculating and providing cost estimates or even bringing USB sticks to AM systems to have files 3D printed.
To make their job easier, most companies often use simple tools like Microsoft Excel to examine data or apps like Zapier to relay data from one machine to another. This stage is often referred to as native automation.
As it requires a combination of manual tasks and simple tools, engineers can still encounter issues when it comes to processing information such as 3D printing requests, file conversions/repair, or project status update.
To address this issue, they often move to “basic or simple automation” and integrate a software tool that can handle multiple steps and applications. In this vein, on the market, one can find AM workflow software that can help engineers to automatically handle ordering, customer requests, rule-based automation for production scheduling, or even distribution of files to specific 3D printers for production.
Beyond these single steps, it is possible to integrate a software solution that automates the whole process from the beginning to the end while enhancing processes over time. Such software tools often require the use of artificial intelligence, and consists in the creation of a seamless digital thread (smart automation) to facilitate visibility and decision-making. This stage of smart automation also brings a certain connectivity and helps create a unified environment for AM with better production planning, traceability and standardisation.
The fourth stage in software automation is certainly the most mature one as it combines the two previous phases with Machine Learning (ML): hyperautomation.
As you may know, once you’ve got a software tool that is based on AI and ML, not only can tasks automatically be optimized, but the software tool utilized here automatically enhances its technology with the increasing amount of data processed.
This stage necessarily involves the question of interoperability that we addressed in a previous issue of 3D ADEPT Mag, Moreover, it should be noted that its integration within a factory is often highly individualised and based on the company’s manufacturing strategy. At this level, the first advice one can give companies is to go for a software platform that is scalable and that is compatible or can easily be adapted with all their platforms and systems.
What are therefore the challenges manufacturers want to address through dedicated automation strategies?
It goes without saying that, each of the aforementioned stages comes with a number of benefits once the appropriate software automation strategy is implemented. As most manufacturers are currently trying to achieve series or mass production with their AM systems, they often try to implement a seamless digital thread or a hyper automation strategy within their production environment.
The fact is, they often mention “investment costs” as one of the hurdles that slow down the integration of an automation strategy but reality shows that they do not often know the digital capabilities they aim to reach.
The first step in understanding what these digital capabilities are, often comes down to recognizing the typical constituent components of additive manufacturing:
- Design & simulation stages;
- Print properties selection;
- Print preparation and orientation;
- Managing in-print queue;
- Initiating printing;
- Removing parts from the 3D printer;
- Post-processing; and
- Finished part retrieval.
“A good example of a specific use case is build preparation for laser powder bed fusion. There are many important factors like part orientation, support generation, and parameter assignment. A mistake can easily be made, and a failed print job can cost tens of thousands of dollars. Oqton [for instance], provides customers with the opportunity to create build preparation templates. We capture their knowledge and experience and couple it with our AI algorithms to automate the complete process. In this way, we speed up the process and lower costs while substantially decreasing human error and improve reproducibility. When you reduce dependency on key operators, this also means that more people can program autonomously. You are no longer at the mercy of one key person’s holiday schedule or them falling sick. This also enables full end-to-end traceability, especially in regulated industries where full documentation is vital, such as medical and aerospace. Ultimately, we see clients increase machine efficiency by faster programming and using advanced manufacturing equipment like welding robots and industrial 3D printers”, Schrauwen notes.
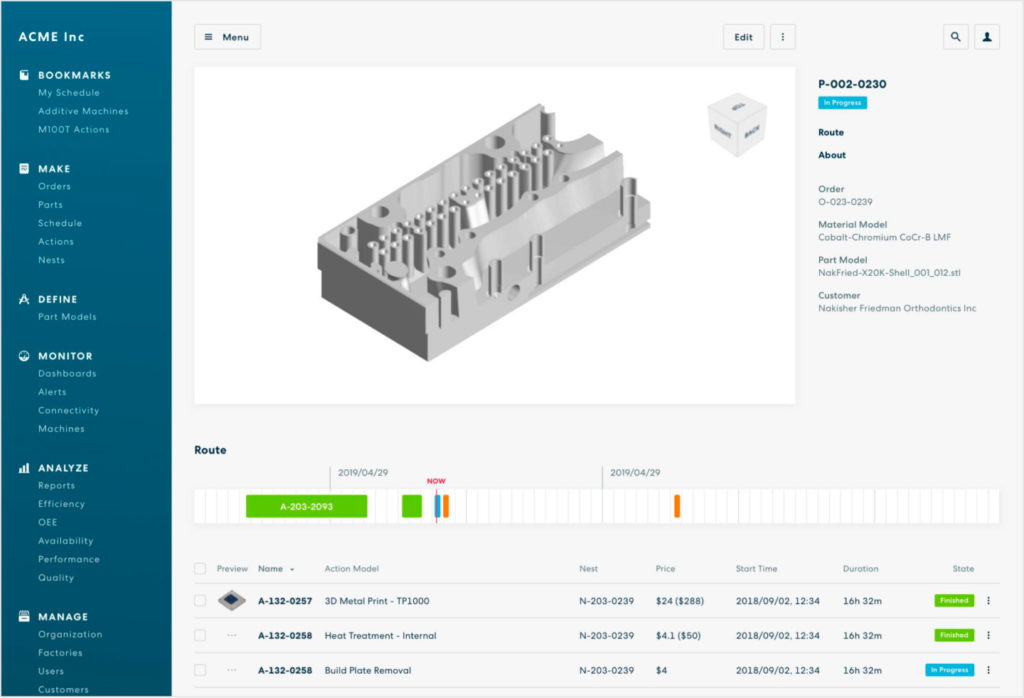
Apart from determining the right digital capabilities one aspires to, it is important to take into account the implementation of an automation strategy with regards to closed AM systems. Although it requires a dedicated article, that’s something we have partially addressed in the exclusive feature “What does “openness” truly mean in a metal AM system?”.
What does the future hold for software automation?
As additive manufacturing advances, it becomes difficult to discuss automation strategies at the software level without any word for its influence on machines. In this vein, let’s note that exploring strategies for advancing automation in post-production processes, as well as automation in 3D printing order and production management should currently be on top of manufacturers’ agenda.
At the software level, which is the heart of this feature, design automation and AM data sharing automation are often mentioned as the key trends that will drive the development of automation solutions.
Design automation for AM
It is no secret that AM helps to reinvent the design of parts and products in different application fields. Despite the design freedom that AM allows, most of the time, AM parts are designed manually. This can be explained by the fact that designing requires a lot of expert knowledge. Complex-shaped parts in particular, can often be an effortful process, both for novice CAD users and experienced designers, hence the need for design automation as an enabler for AM.
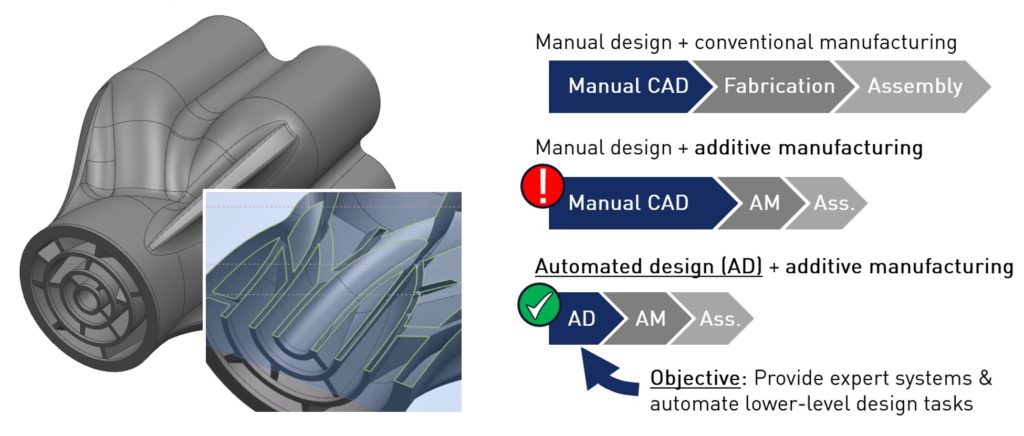
Let’s mention an example shared by researchers from ETH Zurich as an illustration. As part of a project, researchers from the Swiss university demonstrate the potential of design automation by implementing applications in different areas.
“The basic idea is to simplify and accelerate the design phase by providing expert systems that automate lower-level design tasks. Design intent and goals are specified on a high level of abstraction and automatically translated into optimized geometries while taking into account manufacturability restrictions of AM”, they said, speaking of the above image.
Another interesting example saw the development of computational procedures to automate the design of additive manufactured flow components such as hydraulic manifolds. “A designer specifies pathways of flow channels and manufacturing restrictions for AM. Based on these user inputs, algorithms automatically generate a production-ready 3D part geometry that can be used to fabricate prototypes or to conduct numerical simulations. Benefits for the product development include the shortening of iteration loops, the comparison of different production scenarios, and the cost-efficient customization of parts for small lot sizes”, the report reads.
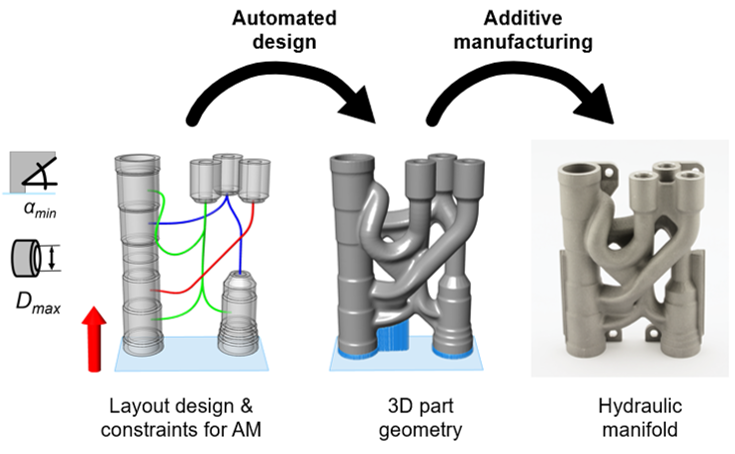
While these examples may be theoretical, it should be noted that, to create digital end-to-end workflow in AM, AM users across vertical industries currently partner with software companies that can help them reduce the time to design tools from hours to minutes.
On the other hand, one notes the increasing number of collaborations between machine manufacturers and software providers to advance this specific area of manufacturing.
AM data sharing automation
The more technology providers create integrated, interoperable 3D printing workflows, the more open Application Programming Interfaces (APIs) are being used to enable connectivity between systems. This increasing use of APIs is a key trend that currently drives AM data sharing automation.
Simply put, an API is a software intermediary that allows two applications to talk to each other. For those who do not know, each time you use an app like Facebook, send an instant message, or check the weather on your phone for instance, you’re using an API.
3DControl Systems, AMFG, or even Dyndrite have been collaborating with machine manufacturers such as HP to enhance such connectivity between systems and expand the use of data. The more APIs-based collaborations will be formed, the more the gap between hardware capability and software enablement will be filled.
“Admittedly, data preparation – build and process data is notoriously difficult to extract, analyse, visualize and share. Every machine manufacturer has its own standards and protocols. End users and the industry would benefit a lot if all manufacturers would openly share data through common protocols and APIs”, our expert notes.
A big shift in focus from hardware to software
However, moving forward the “trends coming around the corner include, a big shift in focus from hardware to software, which includes the move to cloud-based platforms, and the use of AI to automate that process.
IoT connected machines produce lots of data, which in turn requires machine learning AI as the only way to process all that information. Operations will therefore rely more and more on automation to manage their workflows. How to upskill their current workforce to take on new work responsibilities and work with automation is, therefore, both a coming trend and a challenge for the industry at large.
We also see a shift in manufacturing from using 3D printing just for prototyping towards its use within actual production.
Nevertheless, no matter what trend is driving the development of automation solutions in AM right now, the first step for industrials consists in determining at what stage of the journey they are.
This exclusive feature has first been published in the 2021 July/August Edition of 3D ADEPT Mag.