Artificial Intelligence (AI) is part of these advanced technologies that promise massive leaps forward in productivity, environmental friendliness and quality of life, yet confusion around the technology is stronger than ever and dampens its effective integration in manufacturing. Where is therefore the place of AI in a manufacturing sector covered by Additive Manufacturing technologies?
The current artificial intelligence market is difficult to quantify. Although the term is generally understood as the simulation of human intelligence in machines, it should be noted that the term raises confusion with a variety of other segments, including machine learning. Furthermore, experts on the field do not agree with a single definition.
“AI can be seen as an umbrella term, and its scope is incredibly vast. At AMFG, we see AI as a technology that will enable AM machines and systems to be ‘smart’ enough to carry out core operational tasks both effectively and efficiently.
But achieving this requires machine learning. Machine learning is a form of AI and, as the name suggests, it is the idea that machines can learn from data to solve problems and execute tasks – without manual intervention”states Keyvan Karimi, the CEO & Founder of AMFG, that we invited in this segment alongside Additive Flow’s CEO & Co-founder Alexander Pluke as well as Ai Build’s CEO and co-founder Daghan Cam.
If Pluke agrees with the fact that machine learning is a subset of AI inspired by scientific understanding of how humans learn, he outlines that he prefers “a broader definition of AI (and in this sense ‘narrow’ as opposed to a ‘general’ AI as depicted in sci-fi) – where a computational approach can deliver results or recommendations normally completed by human intelligence. In this sense, smart algorithms written by humans that can act ‘intelligently’ – for example evolutionary algorithms and generative approaches, as well as complex classical algorithms that pass the likely subjective threshold of ‘intelligence’, and substitute human activity, could be labelled ‘AI’”.
Nonetheless, AI’s nascent stage of development makes it difficult to understand and to define all the contours of the integration of this technology but also to determine all its applications.
This article serves as an overview of existing efforts by companies which have attempted to discuss the use of AI in AM.
Adapting to process changes
First and foremost, even though AI promises to disrupt the manufacturing environment as we know it, the main issue is that organizations don’t appear to be on the same page about how they want to implement AI. Until today, statistics are not clear about the number of companies that regularly use AI in their production environment.
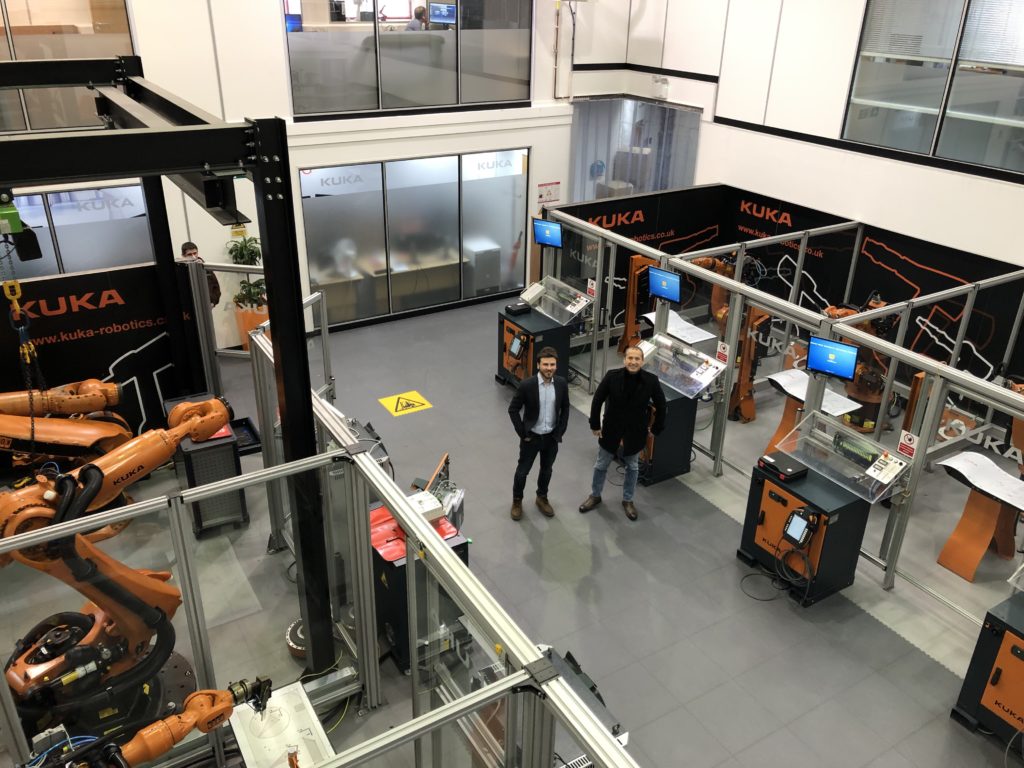
For Ai Build’s Daghan Cam, the problem with this uncertainty in the manufacturing landscape is that not only AI is misinterpreted but companies do not answer the right questions:
“AI is sometimes misinterpreted as a black box software that learns and solves any given problem. That is the definition of Artificial General Intelligence which doesn’t exist today, and most probably won’t exist in the next 10 years. What we have very commonly available instead is narrow forms of artificial intelligence which are extremely powerful in solving specific sets of problems.
In order to leverage these scientific breakthroughs, application developers in manufacturing like in any other industry must be crystal clear about two questions:
- What exactly are we trying to solve with AI?
- How are we going to source the specific type of data that is required to solve that problem?”
Well, it makes sense. How can we address a challenge if that challenge itself is not well defined? In other words, AI will remain a buzzword if there is no upstream strategy.
Research shows that manufacturers that have been able to define a clear strategy have leveraged AI for quality checks, management in smart factories, creation of more reliable designs, reduction of environmental impact to name a few examples.
Is AI a Must in AM?
Despite the media hype and advantages of 3D Printing, there are still a number of reasons that prevent companies from using the technology: financial considerations, speed and the quality of the final part that (does not always match) or requires further investments to match the quality of traditional manufacturing processes like injection moulding.
Interestingly, AI greater potential lies in opening new doors in manufacturing and when it comes to AM, a wide range of cases can be explored.
First, AI allows for a new way of thinking about software.
“Any software driven technology that doesn’t use AI is doomed to fail and will be replaced sooner or later. There is a big responsibility on the machine manufacturers and software developers to work together on redefining the boundaries of additive manufacturing with new releases of their products in order to stay relevant . If a machine is able to bring practical or commercial value to its customers by using AI, it is highly unlikely that other competing products will be able to survive in the market without taking advantage of a similar feature within the next 5 to 10 years depending on the novelty of the process.
For example, Ai Build introduced computer vision into 3D printing in 2016 for autonomous path planning. At that time 3D printers didn’t have cameras embedded so we built a solution from scratch to develop our machine learning algorithms. Nowadays most industrial 3D printers are shipping with built in cameras because of the increasing demand from the market. This is great news because now we are able to work with innovative partners to develop computer vision solutions for different platforms and use cases. If we project 5 years forward, we don’t think any major industrial 3D printers will exist without basic computer vision capabilities like automatic fault detection, because the technology is too useful to be left out, Ai Build’s CEO comments.
Opportunities across the workflow, from design, to production and Quality Assurance (QA).
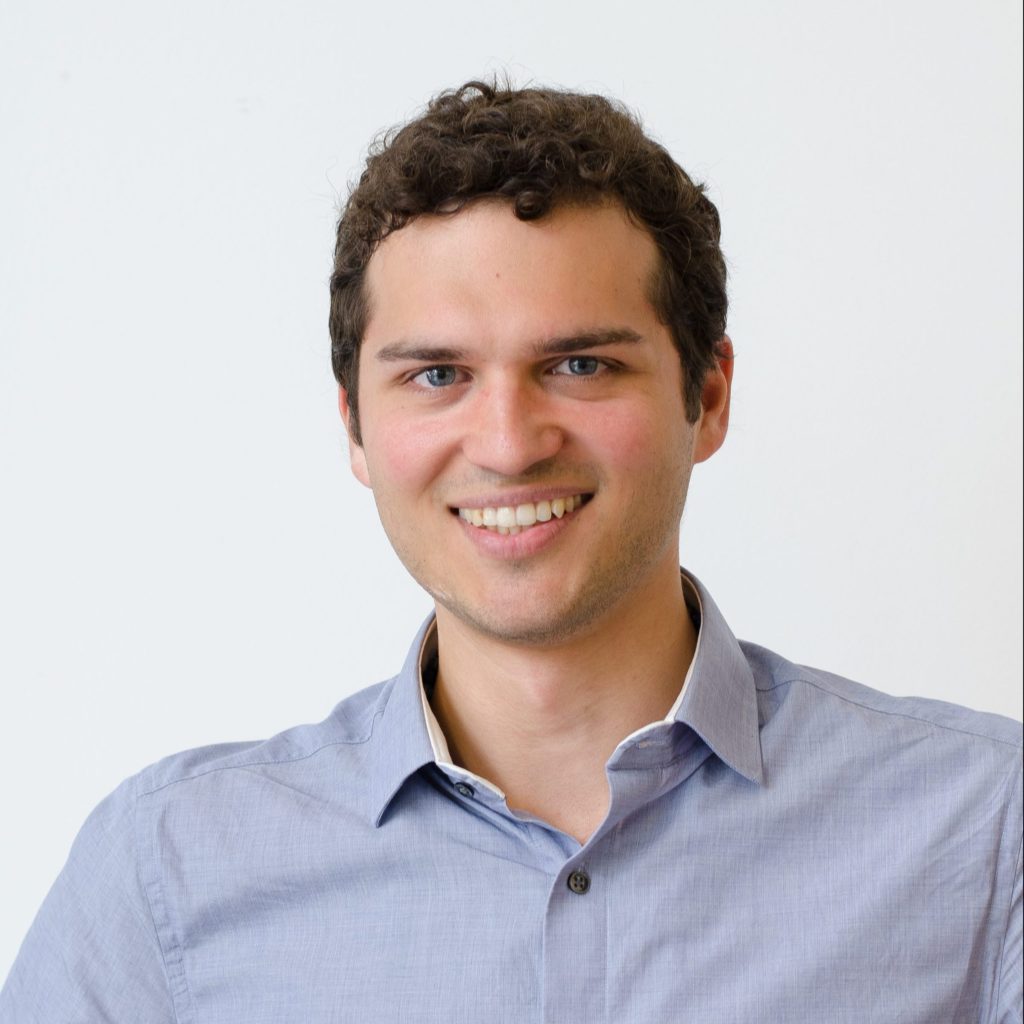
According to Additive Flow’s Alexander Pluke, a number of opportunities for AM in AM can be observed across the workflow, from design, to production and Quality Assurance (QA).
“The complexity of the ‘design domain’ (the possible combinations of design outcomes within a set of variables) is both extremely large, and interdependent. Material quality will affect part performance, which will influence design decisions; and production parameters will affect quality assurance – and quality assurance requirements will (or should!) be reflected in those design decisions… and so on.
Bring in concepts like geometric freedom, and an Additive Flow speciality ‘multi-property’ – where material properties can be tailored within different regions of a geometry – and this complexity gets greater still.
In light of this – rather than asking the question ‘How can AI be leveraged in AM?’, the question should be ‘How can AM be leveraged without AI?’. This is more of a rhetorical question. Without deploying AI-driven tools (in design, in production, in QA, across the workflow), industrial use of AM will not be fully leveraging the opportunity (cost savings, performance, innovation acceleration) we have all heard about.”
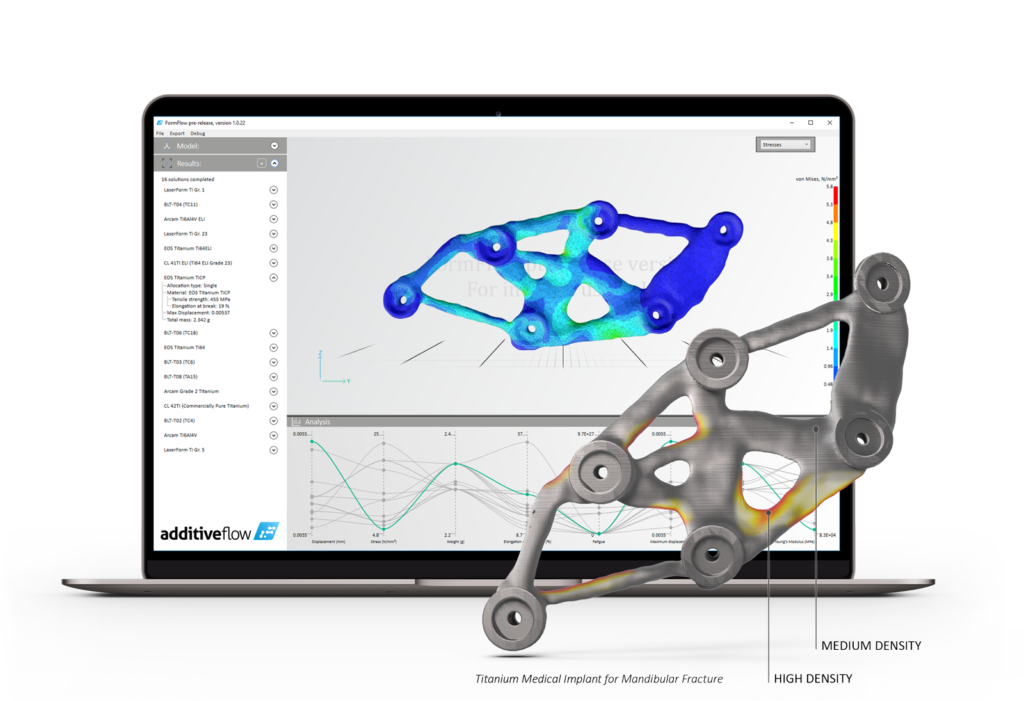
Defect Detection
To avoid repeatable errors, AI could be included in 3D modelling programs through CAD software. Combining AI & 3D Printing to achieve this specific goal would enable the development of tools that will easily find defects and turn the model non-printable into a 3D model.
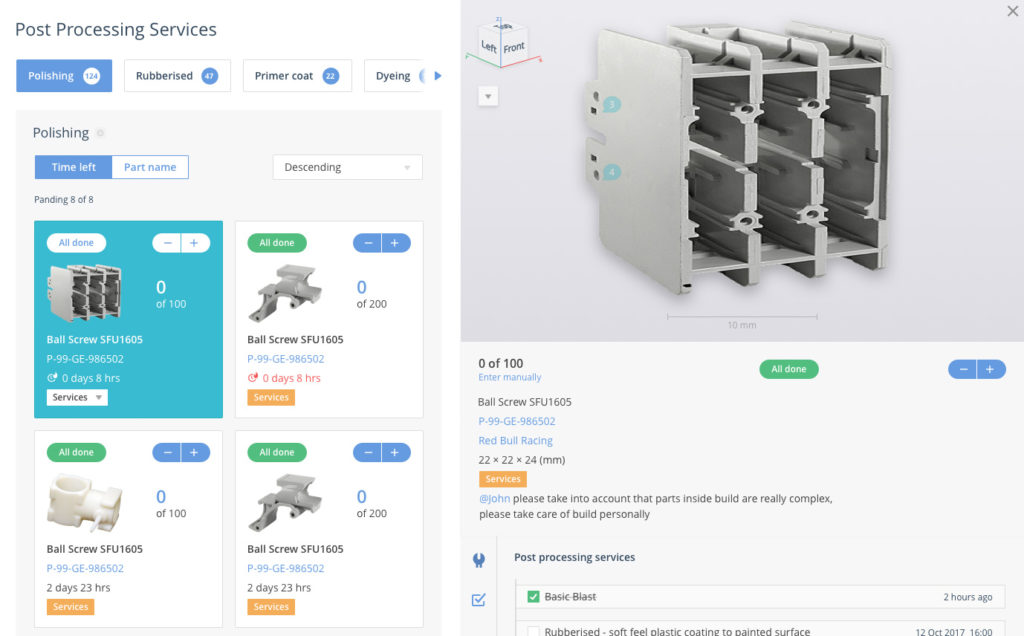
“This enables humans to spend much less time on repetitive, manual tasks and more time on value-adding processes.
Machine learning is one step in this direction. For example, our software is able to provide intelligent material and technology recommendations based on previous data inputs. Our software can also predict part quality and allow users to control the 3D printing process so as to reduce the risk of printing errors. This saves both time and costs.
Additionally, as AM moves to the production of end-use parts, a vast amount of data will be needed to optimise processes. AI will play a role in leveraging this data to achieve faster production and optimal results, so that companies can manage the 3D printing process more effectively”, says Keyvan Karimi from AMFG.
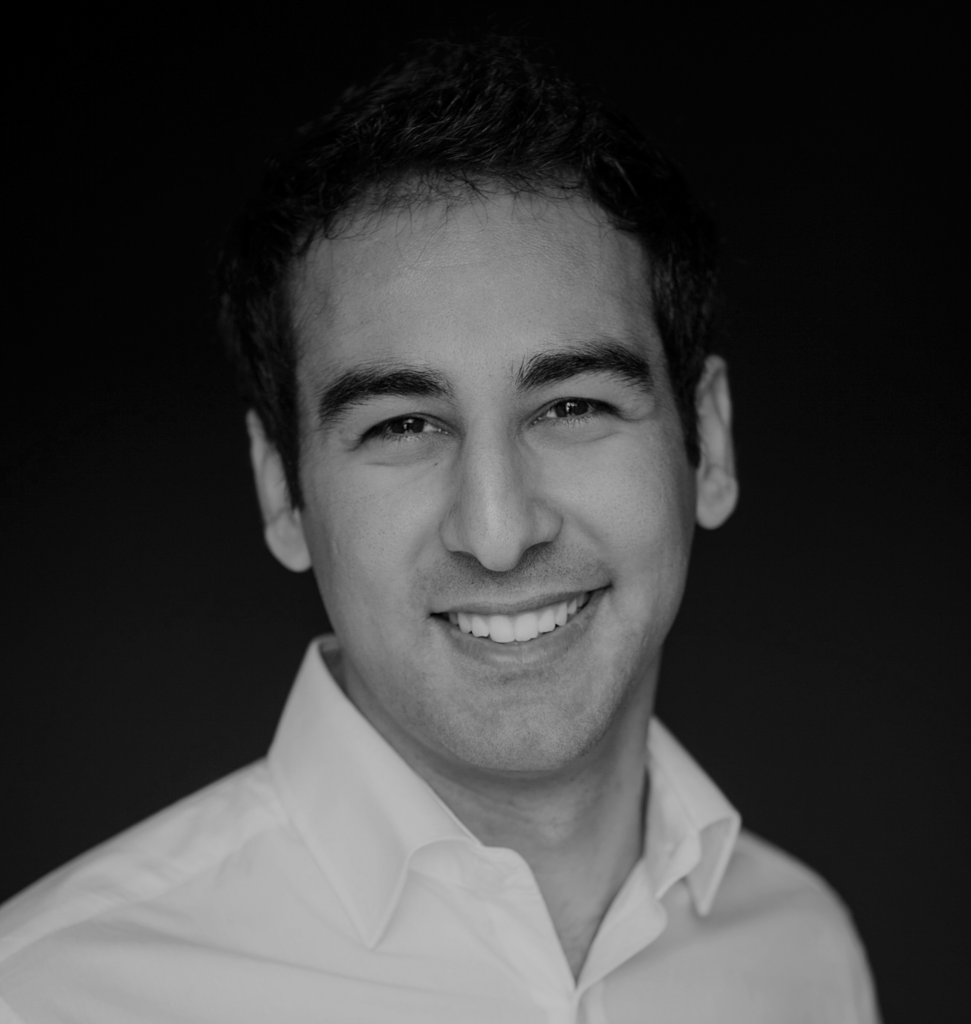
Creating new materials with new properties
The aforementioned examples show an intelligent use of AI before and during the printing process. It should be noted that not only a real-time control by an AI-driven tool can reduce time and material waste, but the technology can also enable the creation of materials that are stronger, lighter, more flexible, and less expensive to produce.
In this specific case, machine learning is often harness to discover these new forms of materials. Materials scientists just need to enter the desired properties they are looking for into a program and algorithms will predict which chemical building blocks can be combined at a micro level to create a structure with the desired functions and properties.
“Data, data, & data!”
In light of these examples, there is no doubt AI and AM must work together. Even though, there are still a few numbers of companies that actually leverage AI for AM. It is hard to say that the market still suffers from a lack of awareness when improvements are seen across the most important fields of the production chain (software, 3D printers and materials).
However, for the three companies that take part in this segment, the most important challenge that slows down the integration of AI in AM is data.
“The best optimisations need the best data sets, and open access to data within this sector is not (yet) prevalent”, explains Pluke.
“One of the biggest challenges is the high level of complexity required to develop AI specifically for 3D printing. Large volumes of data are needed and extensive testing and experimentation done to ensure that the machine is ‘learning’ correctly.
Additionally, AM as an industry is still very much in the early days of integrating 3D printing and AI. Developments for these two technologies are largely happening separately”, adds Karimi.
How do the participating companies approach AI and AM?
As you may have noted, we have invited three UK-based companies to discuss this topic: AMFG, Additive Flow and Ai Build.
AMFG is a software company that provides an end-to-end workflow management solution for autonomous AM. Keyvan Karimi, the CEO & Founder of the company was the main spokesperson in this segment.
The company develops an MES software that integrates elements of machine learning and AI at three levels:
- The ability to analyse the printability of 3D files. AMFG’s software allows for the verification of the 3D printability of a file. As mentioned earlier, the integration of AI in a software might easily find defects (therefore remove the risk of printing errors) and turn the model non-printable into a 3D model. AMFG’s software can also estimate production times.
- The second area covers predictive maintenance – i.e., being able to predict the quality of 3D-printed parts prior to production. AMFG’s software makes it possible to ensure quality throughout the production process. By predicting part orientation for instance, it is possible to determine how the part will be produced.
- Finally, “the third way in which we aim to use AI is through the automation of key process steps across the entire 3D printing workflow”, declares the company.
Additive Flow is another UK-based software company that aims to solve digital workflow issues that currently prevent AM users from unlocking the potential of manufacturing technologies during the pre-production stages of the process chain. Alexander Pluke, CEO and co-founder has shared the company’s expertise on this topic.
To demystify complexity about AI and AM, Additive Flow bets on a system wide approach. The company develops a software named Formflow, which can take in data from across the workflow to put the right material, with the property in the right place.
“Some aspects of the design spaces we are presented with are best suited to neural network approaches, while others are best met with more classical algorithmic techniques. For example, using generative algorithms to optimise exponential variables and allows our users discover new high performing results by revealing solutions that would have otherwise been hidden. Neural networks have provided us some powerful results computationally, and have been useful when considering optimisations that rely on data-structures less digestible by classical algorithms.
We are careful not to ‘do AI for AI’s sake’, but with it, Additive Flow’s software is able to allocate materials, processing parameters, and localised structures simultaneously with geometry – unlocking value in AM in ways that would otherwise be possible”, emphasizes the company.
Lastly, Ai Build developsadditive manufacturing software & hardware for factories of the future. The 3D printer manufacturer’s real-time inspection technology helps to detect and prevent 3D printing errors thanks to artificial intelligence. Daghan Cam, the company’s CEO and co-founder was our guest in this dossier.
The company first came to our radar two years ago when its technology was displayed in the Sculpture gallery of the Victoria and Albert Museum. Over time, we realized it also stands out from the crowd thanks to its AiSync software.
“One of the foundational decisions we made in AiSync software to enable an AI driven additive manufacturing process is to deliberately avoid using gcode files to describe a toolpath. This was very counterintuitive because all 3D printers and CNC machines on the market that we are aware of use gcode or a brand specific gcode-like language to drive the motions of machines. Gcode would work fine in a perfectly deterministic world where the whole process could be predicted with high accuracy, but unfortunately the additive manufacturing process in real life is far from perfect. We are not able to predict the physical form of 3D printed parts precisely because even the tiniest change in the build volume during production can lead to catastrophic deviations in the additive process. Even if we could fully control the environment and if there was a powerful simulation engine to precisely predict material behaviour 99.9% of the time in one layer, the success rate of the overall prediction on a typical part with thousands of layers that support each other would still be less than 30% for statistical reasons.
This was the key insight for us to abandon gcode and develop a completely new machine control process from scratch that is more resilient. In AiSync software we use a combination of offline and online optimization methods to control the actions of 3D printers. Instead of slicing a design and uploading static gcode files onto machines, AiSync analyzes a design with powerful computers on the cloud and sends optimized directives to the machines in an abstract format. These directives are then interpreted and turned into machine level instructions bit by bit on the fly by another computer that is located on the edge which has real-time access to sensor data from the machine. This double optimisation infrastructure enables us to take full advantage of AI algorithms by combining the strengths of cloud supercomputing for high level tasks like path planning and edge computing for time critical tasks like fault detection and quality assurance”, describes the company.
To sum up…
This dossier shows that despite the perfect match between AI and AM, we are still very far from a perfect integration of both processes in manufacturing. Indeed, the biggest strength of AI is also its major weakness: data. To overcome this challenge, both standardization and collaborations between stakeholders are necessary to enable the right integration of AI in AM processes.
This dossier has originally been published in the May – June Issue of 3D ADEPT Mag.
Remember, you can post free of charge job opportunities in the AM Industry on 3D ADEPT Media or look for a job via our job board. Make sure to follow us on our social networks and subscribe to our weekly newsletter : Facebook, Twitter, LinkedIn & Instagram ! If you want to be featured in the next issue of our digital magazine or if you hear a story that needs to be heard, make sure to send it to contact@3dadept.com