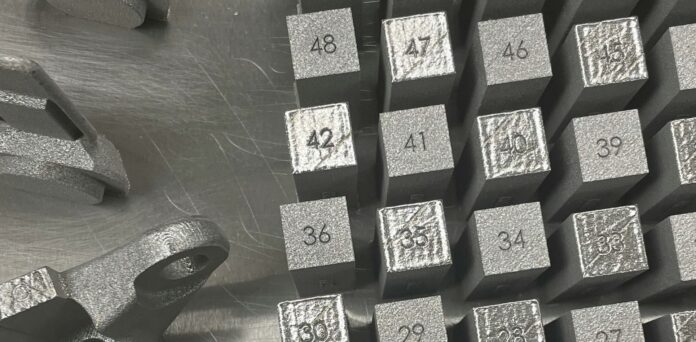
Software provider Senvol recently demonstrated a machine learning approach to material allowables development that was shown to be more flexible, cost-effective, time-effective, and equivalent to the conventional (in this case, MMPDS) approach to material allowables development.
This demonstration was done as part of a U.S. Government contract W911NF-20-9-0009 that the company has recently been awarded.
The program focused on demonstrating the approach using a 17-4 PH Stainless Steel material processed via a powder bed fusion AM machine.
AM is starting to enable lightweight and rapidly produced designs that are revolutionary to various defense and commercial capabilities and applications. These benefits cannot yet be fully realized due to the time and high cost of allowables development.
The high cost stems in large part from the fact that material allowable development requires an enormous amount of empirical data to be generated, at a fixed processing point, meaning that all of the empirical data must typically be regenerated from scratch every time there is a major change in the process. This results in an AM process that is not only costly and time-consuming to implement the first time, but costly and time-consuming to maintain in the long-run when there are inevitably changes to the AM process.
Hector Sandoval, who reviewed the AMMP program technical approach and test results, adds, “I have experience developing material allowables using traditional methodologies. The current process works well, but there are some limitations. It was exciting to support the AMMP program by reviewing the technical approach, test results, and final presentation. It was great to get a first-hand look at the potential of leveraging a machine learning based approach to help establish material allowables.”
The Senvol ML software supports the qualification of AM processes and was used in the program to develop statistically substantiated material properties analogous to material allowables. Furthermore, it did so while simultaneously optimizing data generation requirements. Important to note is that the software is flexible and can be applied to any AM process, any AM machine, and any AM material. Also of note is that this project did not develop true allowables. Due to budget and programmatic constraints, the project team had to make several simplifying decisions.
Senvol President Zach Simkin comments, “The use of machine learning for additive manufacturing process and material development is very mature. This has been adopted by industry and is the low-hanging fruit. The use of machine learning specifically for material allowables development, however, is still a work in progress. I’m pleased that we have now made two successful demonstrations of the machine learning approach to allowables – once in this program using a metal alloy and comparing to MMPDS, and once in a prior program funded by America Makes that used a polymer material and compared to CMH-17 – but more research is needed. The upside is tremendous, and we look forward to continuing to partner with the Government and industry to advance work in this area.”
Remember, you can post free of charge job opportunities in the AM Industry on 3D ADEPT Media or look for a job via our job board. Make sure to follow us on our social networks and subscribe to our weekly newsletter : Facebook, Twitter, LinkedIn & Instagram ! If you want to be featured in the next issue of our digital magazine or if you hear a story that needs to be heard, make sure to send it to contact@3dadept.com